On Cognitive Flexibility Theory and its Investing Implications
A helpful framework for building investing expertise by collecting a library of prior cases and maintaining an 'adaptive worldview'
Last week, Cedric Chin of Commoncog generously released his series 'Learning in Ill-Structured Domains' free to the public. While applicable to various fields, the series immediately helped me better understand how we should be looking to build expertise in investing, an area where concepts and real-world instantiations of those concepts almost never map perfectly together.
The below is my very simplistic summary/interpretation of Cedric's writing (without having done the primary research he's clearly done) and initial implications for Central Tendency and my investment process more broadly. If it's not clear: Almost nothing below comes from my original thoughts. I highly recommend you read Cedric's series directly here, as well as his other work (which I've followed for some time). He has a great way of distilling complicated concepts into usable ideas.
Structured vs. Ill-Structured Domains
Most traditional learning is concept-oriented - Individuals are taught or seek frameworks/principles that are often memorized, utilized, and tested, with case studies occasionally provided to supplement learning. Provided case studies help illustrate the concept, but are ultimately discarded in favor of retention of the concept itself. A lot of us are tuned to seek knowledge in this way.
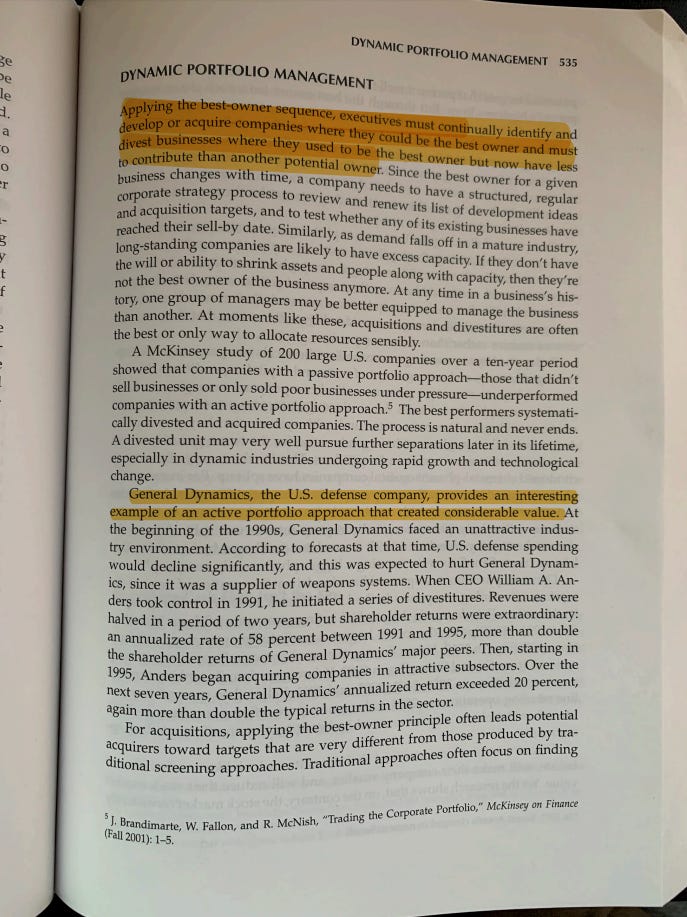
There's nothing inherently wrong with this type of learning. In fact, it is extremely relevant for particular fields called structured domains, defined as areas where concepts always show up in practice in the same way they do in theory. An easy example of a structured domain is math, including concepts such as calculus. Once you learn the core rules of calculus, they can be applied in all scenarios in which you encounter calculus regardless of situational factors such as the time of day, geography, etc.
However, much of what we encounter in our day-to-day lives is not so structured, requiring us to constantly adapt to novel situations we haven't seen before. Learned frameworks do not help us consistently understand or accurately predict exactly what is going to happen in every circumstance.
Such fields are called ill-structured domains. In contrast to structured domains, these fields are characterized by the fact that, while concepts exist, they are not generalizable in every context. Investing is a clear example of this type of field.
Take a fundamental, incredibly important investing concept: Intrinsic value. This refers to the value of what a business should be worth, rather than its current valuation. Investors typically define a business as undervalued when it trades below its intrinsic value and overvalued when it trades above its intrinsic value, making this a core investing concept.
A novice or layperson may have a conceptual framework of what calculating intrinsic value looks like (likely an analyst inputting numbers into a spreadsheet to get to a precise business valuation), but the reality is that intrinsic value is incredibly difficult to pinpoint and, importantly, the relevant factors for its calculation vary greatly by situation.
The term 'intrinsic value' was popularized in Benjamin Graham's Security Analysis. Graham discusses intrinsic value below:
"We must recognize, however, that intrinsic value is an elusive concept. In general terms it is understood to be that value which is justified by the facts, e.g., the assets, earnings, dividends, definite prospects, as distinct, let us say, from market quotations established by artificial manipulation or distorted by psychological excesses. But it is a great mistake to imagine that intrinsic value is as definite and as determinable as is the market price […] The essential point is that security analysis does not seek to determine exactly what is the intrinsic value of a given security. It needs only to establish either that the value is adequate—e.g. , to protect a bond or to justify a stock purchase—or else that the value is considerably higher or considerably lower than the market price." - Security Analysis (2nd ed.)
As Graham notes, intrinsic value is not precise in the same way as a stock price. It is impossible to exactly calculate, with the security analyst only interested in whether intrinsic value is considerably higher or lower than the current price. Importantly, Graham does not tell us how to calculate intrinsic value. Instead, he notes various facts that could justify intrinsic value (assets, earnings, prospects, or any other variable). The relevance and relative importance of any of these specific factors is entirely dependent on the situation, including the company's growth rates, returns, industry dynamics, time period, etc.
You could try to come up with more specific rules around the calculation of intrinsic value, but these would likely end up being too dependent on context to be useful. Let's take an example that I think most people would agree with: "A company that is higher quality than the overall stock market should have an intrinsic value multiple that is higher than the prevailing market multiple."
But how should we define 'higher quality'? What multiples should we use (EBITDA, earnings, book value, etc.)? What if the current market multiple is depressed/too high vs. history? You could come up with many more questions like this and the answers to each question would vary by situation. It would be extremely difficult to codify the concept of intrinsic value into consistently applicable principles.
The natural question then is: How should one become better at calculating intrinsic value, when the way this concept shows up in reality can vary greatly in each situation? Buffett is an expert at this calculation, and discussed his approach at the 2008 Berkshire Hathaway Annual Meeting:
"We can make a decision in five minutes very easily. I mean, it just is not that complicated. Now, we know about a number of businesses and industries, and there’s a lot of businesses and industries we don’t know anything about. […] But the most important thing is that anything that gets through is in an area of knowledge. And the truth is, if we can’t make a decision in five minutes, we can’t make it in five months.
[...] If we get a call and somebody says either they’ve got a business for sale or — that’s what we’re going to get on the calls — or if I’m reading a paper, or a magazine, or an annual report, or a 10-K, and I look at a price and there’s a significant differential between price and value, we move right then." - Warren Buffett, Berkshire Hathaway Annual Meeting (2008)
Buffett is indicating he can make intrinsic value calculations within five minutes. He doesn't mention complex math equations (although I appreciate Buffett has a great ability to do mental math) nor does he mention predefined rules ("intrinsic value should be calculated in XYZ way each time"). Instead, Buffett compares the business being analyzed to existing areas of knowledge he already has.
Cognitive Flexibility Theory
Cognitive Flexibility Theory ("CFT") provides an explanation of how to build expertise in ill-structured domains like investing, which seems to apply well to Buffett's approach. The theory has two central ideas:
Given concepts are not generalizable in ill-structured domains, prior cases are as, if not more, important than concepts themselves. Fragments of prior cases are referenced to help analyze novel situations (which are frequent in ill-structured domains)
Prior and future cases should be understood to have emergent properties and thus should be expected to be inherently unpredictable, irregular, and indeterminate. As a result, cases should not be simplified to generalizable principles. Said differently, there is no one root concept that fully explains an event (this kind of thinking is called an 'adaptive worldview')
CFT indicates that concepts are only useful in their practical application in the real world. Its two central ideas go hand-in-hand - A collection of relevant prior cases helps color an individual's understanding of the concept itself, but it should be understood that the outcomes of each prior case were dependent on context (just because something has happened in the past, doesn't mean it will always happen in the future).
Using our previous example, we can try to conceptualize intrinsic value as much as we want, but CFT argues we won't fully understand the concept until we study real examples of both undervaluation and overvaluation, analyzing the differences and similarities of prior cases as well as instances where intrinsic value was ultimately irrelevant. Over time, this approach should allow us to get better at pulling on the relevant fragments from our existing knowledge to make more informed decisions. I believe this is what Buffett is doing.
It follows then that in order to gain expertise in ill-structured domains, we must identify and analyze as many cases as possible to draw upon and help inform understanding of various concepts. We also most constantly maintain an adaptive worldview.
Kind and Wicked Learning Environments
To be clear, prior cases need not be from 3rd parties, but can also be from your own experiences. The important thing is that regardless of where they come from, prior cases should have identifiable and reviewable feedback for any decisions made, such that we can attempt to discern (to whatever extent possible) whether decisions made led to good or bad outcomes and whether such outcomes were based on luck or skill.
Environments where such feedback is readily available are called 'kind' learning environments. By providing individuals with an understanding of whether decisions are leading to desired outcomes, expert intuition can be built over time. In contrast, 'wicked' learning environments are those without such feedback and often lead to hubris and incorrect intuition as individuals can't identify when they're making mistakes (leading them to make the same mistakes over and over).
An important nuance - Just because a field is ill-structured, doesn't mean you can't create a kind learning environment. For example, in investing, a more kind learning environment can be built by regular reviews of prior buy/sell/pass decisions. Are your investments performing for the reasons you thought they would? Are your sell decisions adding any value? Are you passing on too many good opportunities?
Reviewing this feedback should help you understand to a greater extent if your decision making has been successful and if outcomes have truly been based on skill. Ultimately, this should drive individuals towards expertise.
Investing Implications
I believe the above has profound implications for the investing process. There are two things I want to make sure I'm doing going forward:
Building a better set of prior case studies in line with CFT that I can use to drive understanding of concepts. I don't currently feel I have enough prior cases that I understand and can reference when making decisions. By building this set, hopefully I'll be more likely to say, "this reminds me of when..." when encountering a concept, rather than simplifying the concept to theory or non-generalizable principles. These cases will likely increasingly be posted to Central Tendency as I encounter them
To be clear, I still think it's very important to constantly be reading about and analyzing new investing ideas (which I'll continue to post) - This is the 'skill' we're honing when working towards investing expertise. However, we want to make sure we're getting better at this skill and, unfortunately, feedback periods in investing can be extremely long (often years). It's definitely worth learning from the experiences of others to incorporate feedback more readily
Cedric is building his own case library at Commoncog, which he's also generously made free to the public
Creating a more kind learning environment within my own day-to-day investing. Central Tendency is a good way to track initial buy/sell/pass decisions, but there's still so many decisions that I don't track. I need to do a better job of clearly highlighting the thesis for an idea and tracking against that thesis (not just the stock price), making sure I do post-mortems for ideas and following up on any sales over time, and somehow tracking the multitude of ideas I ultimately don't choose to write-up. I'm still thinking about the best ways to implement all this, but creating better feedback loops should help refine my investing process over time
While I'm aware none of this is necessarily ground breaking (using case studies and better decision tracking for investing isn't revolutionary), I don't think it's something enough investors think about or include in their process. I'm looking forward to tracking the results.